AI at the Edge
January 27, 2023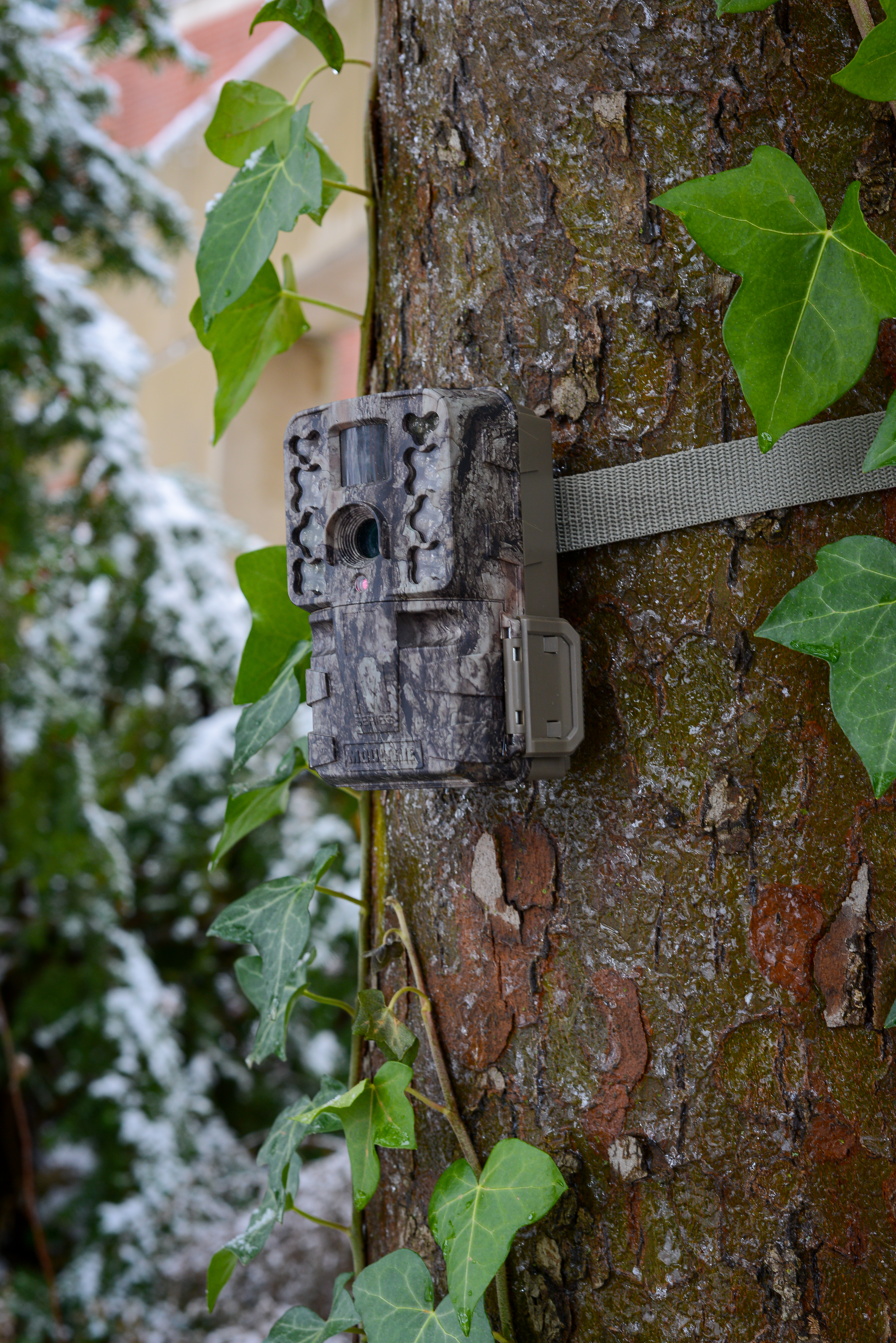
New trail camera holds potential to transform how we study ecosystems
Since the 1880s when the first trail camera was invented, wildlife researchers and hunters have been trekking out into the fields to check on their camera. Now they bring back the camera’s SD card to their computer just to sift through millions of photos, mostly blank, to see if, for example, a wolverine happened to saunter by the camera in the past few days.
This slow and tedious method of identifying wildlife will soon become old school with the creativity of scientists like Luke Sheneman of University of Idaho’s Research Computing and Data Services (RCDS). Instead, what if we could use artificial intelligence (AI) to automatically identify animals and transmit an identification signal via satellite to a computer?
This is called “AI at the edge†— deploying AI at the site of detection and transmitting results in real time. From radiologists to city planners, many are invested in the idea. But trail camera manufacturers haven’t commercialized it in their products yet.
The task of creating an AI prototype isn’t simple. But after gathering 1.2 million trail camera training images and waiting 3 weeks for the AI to sift through them all, Sheneman has trained a machine learning model that can identify 25 species of animals with over 90% accuracy. Sheneman continues to improve its capabilities and accuracy, and the model has quickly garnered interest for a seemingly endless array of possibilities.
The camera started out as part of a project called Wildlife and Humans in Shared Landscapes (WHISL). As drought plagued the western U.S., this project began investigating how humans and livestock interact with wildlife on the landscape. Sheneman’s camera will help the team estimate population sizes and density of wildlife, then alert ranchers through a mobile app if their livestock would be likely to encounter a predator. This is an exciting prospect for ranchers trying to protect their sheep and cattle from predation.
Trail camera images classified by Sheneman's AI model
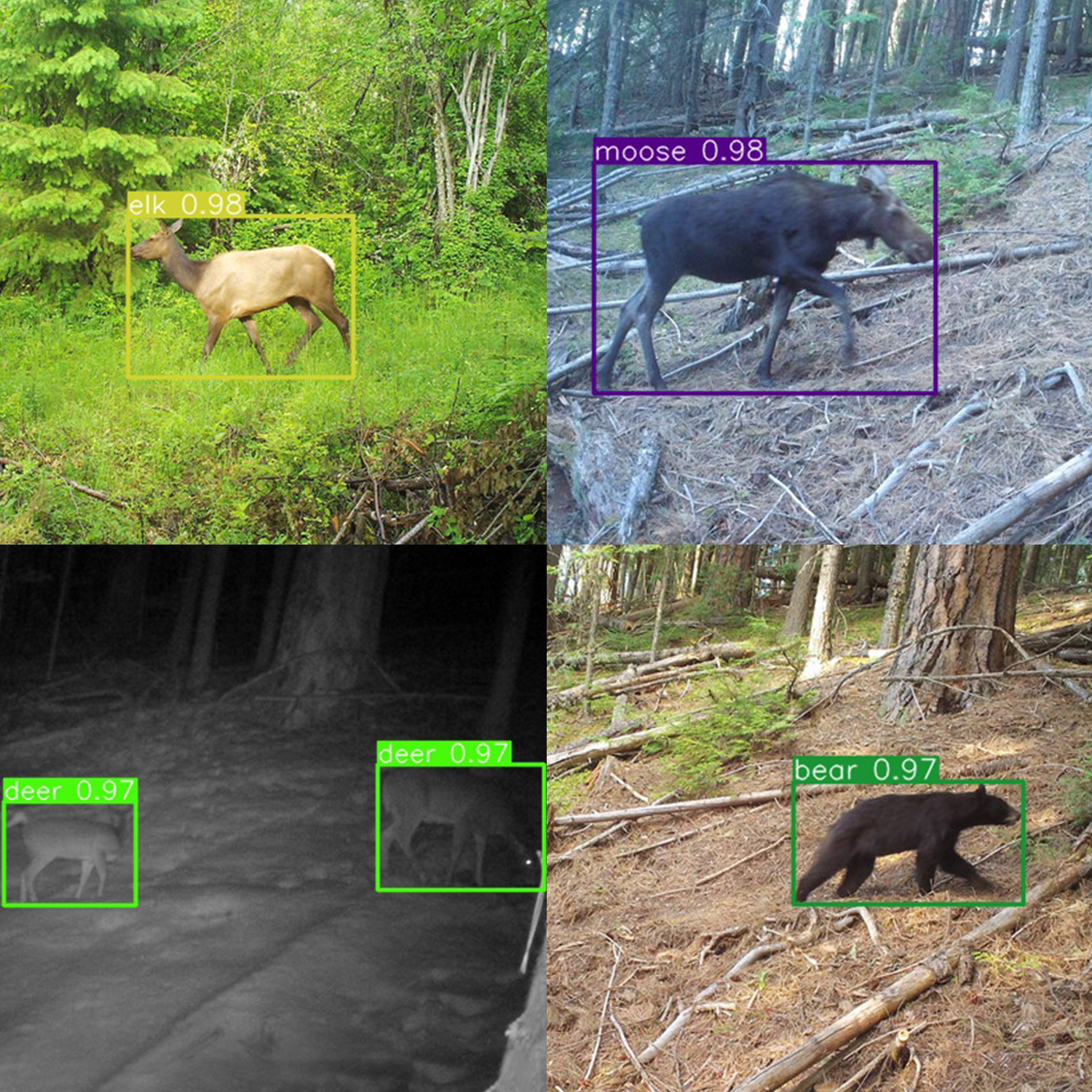
Within this context, the camera’s AI model could also begin to classify and measure edible food sources by, for example, assessing the height, quantity, and greenness of edible grass and forbs. This could be especially useful on landscapes where drones and satellites can’t see through the forest canopy.
The AI-powered camera could even start to classify sounds to detect nearby wildlife outside the camera’s field of view. This would involve incorporating bioacoustic sensors into the hardware so that, as Sheneman puts it, “not only is it watching, but it’s listening.â€
The U.S. Forest Service in Montana is also interested in using the camera in its project to track the movement of lynx in northern Montana. After tagging the lynx with collars, the camera could pick up the collars’ radio signals and relay their location to scientists via satellite.
For agencies that are sifting through tens of millions, if not hundreds of millions, of camera trap photos every year, the idea of having a camera that transmits highly accurate classifications in real time is highly enticing. Idaho Fish and Game (IDFG), Oregon Department of Fish and Wildlife (ODFW) and the U.S. Forest Service in Montana have all expressed interest in this prototype.
U of I and IDFG have been fostering an informal collaboration in this area. While Sheneman’s trained models improve IDFG’s photo analysis workflow, IDFG can provide Sheneman with massive amounts of valuable image data to keep training his AI model to better classify more species – whether that’s animals, plants, sounds, or specific traits of the same species.
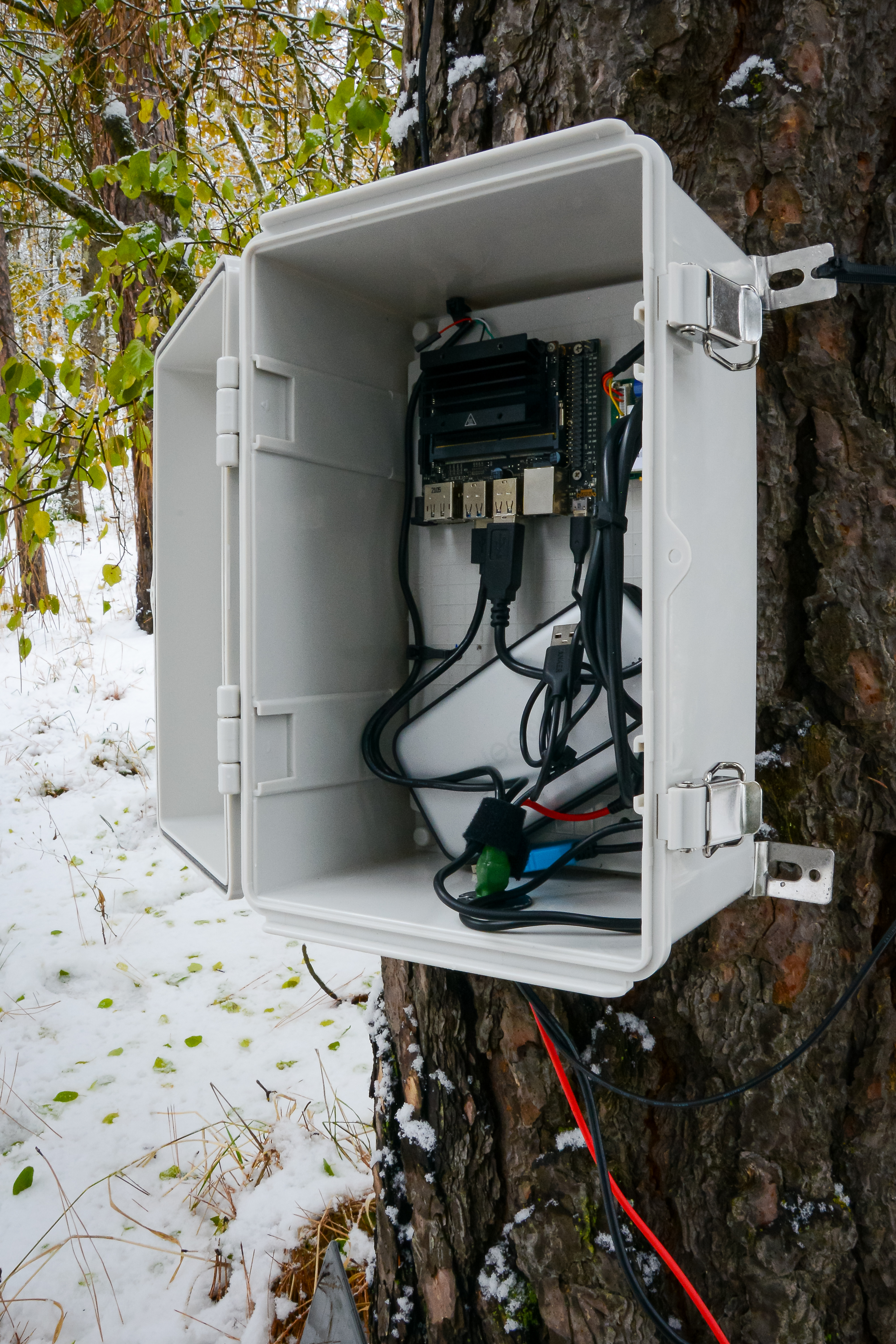
His next step is to classify up to 50 distinct animal species, which alone would require at least five million training images. These images are available to him through his collaboration with IDFG and ODFW, but the work involves long hours of labeling and training the AI system to recognize the species and their states, which could take months on specialized computing infrastructure available through RCDS at the U of I.
For students interested in joining Sheneman’s mission, he’s looking for “a small army of labelers to help.†It only takes one round of intense labeling to build powerful, accurate AI models that can do that work automatically in future seasons.
“Now that we have this computer out in the woods with a satellite connection [and a powerfully accurate AI model], scientists are asking, what else can we get it to do?†says Sheneman.
Article by Kelsey Swenson,
IIDS Scientific Writing Intern